PDF: |
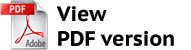 |
Author(s): |
Konnikov E. A., Kryzhko D. A., Pospelova A. S., Zaborovskaya O. V., |
Number of journal: |
4(65) |
Date: |
November 2023 |
Annotation: |
The financial health of commercial enterprises is crucial for the stability and growth of any economy. However, assessing a company’s financial stability can be a challenging task. As the risk of bankruptcy is constantly looming, credit institutions are in constant search for reliable tools to forecast the likelihood of a company’s financial collapse. Traditional risk assessment methods have their limitations, which is why researchers have turned to ensemble methods that combine multiple models to improve forecasting accuracy. By using a variety of models, each with its own strengths and weaknesses, ensemble methods aim to provide more comprehensive and accurate forecasts than individual models. The goal of these methods is to identify potential bankruptcies early on and provide creditors with the opportunity to take necessary actions to effectively reduce risks. This article explores how the implementation of ensemble methods can increase the accuracy of bankruptcy forecasting and provide credit organizations with a powerful tool for assessing the financial position of companies. It is worth noting that the use of machine learning and artificial intelligence capabilities provides significant improvements compared to traditional approaches that rely on limited data processing options. Ensemble methods allow researchers to increase the accuracy of bankruptcy forecasting and provide credit organizations with a reliable tool for assessing the financial stability of trading enterprises. By implementing these complex methods, credit organizations can make more informed decisions regarding lending and investments, which can have a significant impact on the stability of the financial market. |
Keywords: |
bankruptcy forecasting, service enterprises, collective learning methods, ensemble methods, financial stability,
risk assessment, machine learning, artificial intelligence, forecasting accuracy, credit institutions |
For citation: |
Pospelova A. S., Zaborovskaya O. V., Kryzhko D. A., Konnikov E. A. Forecasting the probability of bankruptcy
of service enterprises on the basis of collective learning methods. Biznes. Obrazovanie. Pravo = Business. Education. Law.
2023;4(65):54—63. DOI: 10.25683/VOLBI.2023.65.802. |